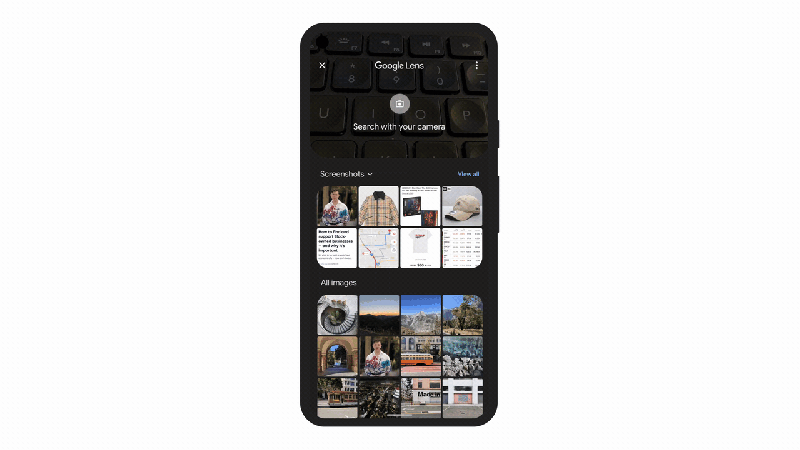
A new GamesBeat occasion is about the corner! Learn more about what comes next.
During a livestreamed occasion today, Google detailed the approaches it is working with AI strategies — particularly a machine studying algorithm named multitask unified model (MUM) — to improve internet search experiences across unique languages and devices. Beginning early next year, Google Lens, the company’s image recognition technologies, will acquire the potential to discover objects like apparel based on images and higher-level descriptions. Around the exact same time, Google Search customers will commence seeing an AI-curated list of issues they should really know about particular subjects, like acrylic paint supplies. They’ll also see ideas to refine or broaden searches based on the subject in query, as nicely as associated subjects in videos found by way of Search.
The upgrades are the fruit of a multiyear work at Google to increase Search and Lens’ understanding of how language relates to visuals from the internet. According to Google VP of Search Pandu Nayak, MUM, which Google detailed at a developer conference last June, could support improved connect customers to firms by surfacing merchandise and reviews and enhancing “all kinds” of language understanding, regardless of whether at the buyer service level or in a investigation setting.
“The power of MUM is its ability to understand information on a broad level. It’s intrinsically multimodal — that is, it can handle text, images, and videos all at the same time,” Nayak told VentureBeat in a phone interview. “It holds out the promise that we can ask very complex queries and break them down into a set of simpler components, where you can get results for the different, simpler queries and then stitch them together to understand what you really want.”
MUM
Google conducts a lot of tests in Search to fine-tune the outcomes that customers eventually see. In 2020 — a year in which the business launched more than 3,600 new features — it performed more than 17,500 site visitors experiments and more than 383,600 excellent audits, Nayak says.
Still, offered the complicated nature of language, concerns crop up. For instance, a search for “Is sole good for kids” quite a few years ago — “sole” referring to the fish, in this case — turned up webpages comparing kids’ footwear.
In 2019, Google set out to tackle the language ambiguity challenge with a technologies named Bidirectional Encoder Representations from Transformers, or BERT. Building on the company’s investigation into the Transformer model architecture, BERT forces models to contemplate the context of a word by seeking at the words that come just before and immediately after it.
Dating back to 2017, Transformer has come to be the architecture of option for all-natural language tasks, demonstrating an aptitude for summarizing documents, translating in between languages, and analyzing biological sequences. According to Google, BERT helped Search improved comprehend 10% of queries in the U.S. in English — especially longer, more conversational searches exactly where prepositions like “for” and “to” matter a lot to the which means.
For instance, Google’s earlier search algorithm wouldn’t comprehend that “2019 brazil traveler to usa need a visa” is about a Brazilian traveling to the U.S. and not the other way about. With BERT, which realizes the significance of the word “to” in context, Google Search offers more relevant outcomes for the query.
“BERT started getting at some of the subtlety and nuance in language, which was pretty exciting, because language filled with nuance and subtlety,” Nayak stated.
But BERT has its limitations, which is why researchers at Google’s AI division created a successor in MUM. MUM is about 1,000 occasions bigger than BERT and educated on a dataset of documents from the internet, with content like explicit, hateful, abusive and misinformative photos and text filtered out. It’s capable to answer queries in 75 languages such as concerns like “I want to hike to Mount Fuji next fall — what should I do to prepare?” and recognize that that “prepare” could encompass issues like fitness instruction as nicely as climate.
MUM can also lean on context and more in imagery and dialogue turns. Given a photo of hiking boots and asked “Can I use this to hike Mount Fuji?” MUM can comprehend the content of the image and the intent behind the query, letting the questioner know that hiking boots would be acceptable and pointing them toward a lesson in a Mount Fuji weblog.
MUM, which can transfer expertise in between languages and does not will need to be explicitly taught how to full particular tasks, helped Google engineers to recognize more than 800 COVID-19 name variations in more than 50 languages. With only a handful of examples of official vaccine names, MUM was capable to discover interlingual variations in seconds compared with the weeks it may take a human group.
“MUM gives you generalization from languages with a lot of data to languages like Hindi and so forth, with little data in the corpus,” Nayak explained.
Multimodal search
After internal pilots in 2020 to see the kinds of queries that MUM may be capable to resolve, Google says it is expanding MUM to other corners of Search.
Soon, MUM will permit customers to take a image of an object with Lens — for instance, a shirt — and search the internet for yet another object — e.g., socks — with a related pattern. MUM will also allow Lens to recognize an object unfamiliar to a searcher, like a bike’s rear sprockets, and return search outcomes according to a query. For instance, offered a image of sprockets and the query, “How do I fix this thing,” MUM will show directions about how to repair bike sprockets.
“MUM can understand that what you’re looking for are techniques for fixing and what that mechanism is,” Nayak stated. “This is the kind of thing that the multimodel Lens promises, and we expect to launch this sometime hopefully early next year.”
As an aside, Google unveiled “Lens mode” for iOS for customers in the U.S., which adds a new button in the Google app to make all photos on a webpage searchable by way of Lens. Also new is Lens in Chrome, readily available in the coming months globally, which will permit customers to pick photos, video, and text on a internet site with Lens to see search outcomes in the exact same tab without having leaving the web page that they’re on.
In Search, MUM will energy 3 new features: Things to Know, Refine & Broaden, and Related Topics in Videos. Things to Know requires a broad query, like “acrylic paintings,” and spotlights internet sources like step-by-step directions and painting types. Refine & Broaden finds narrower or basic subjects associated to a query, like “styles of painting” or “famous painters.” As for Related Topics in Videos, it picks out subjects in videos, like “acrylic painting materials” and “acrylic techniques,” based on the audio, text, and visual content of these videos.
“MUM has a whole series of specific applications,” Nayak stated, “and they’re beginning to impact on many of our products.”
Potential biases
A increasing body of investigation shows that multimodal models are susceptible to the exact same kinds of biases as language and laptop vision models. The diversity of concerns and ideas involved in tasks like visual query answering — as nicely as the lack of higher-excellent information — frequently avert models from studying to “reason,” major them to make educated guesses by relying on dataset statistics. For instance, in one study involving 7 multimodal models and 3 bias-reduction strategies, the coauthors discovered that the models failed to address concerns involving infrequent ideas, suggesting that there’s work to be carried out in this location.
“[Multimodal] models, which are trained at scale, result in emergent capabilities, making it difficult to understand what their biases and failure modes are. Yet the commercial incentives are for this technology to be deployed to society at large,” Percy Liang, Stanford HAI faculty and laptop science professor, told VentureBeat in a current e mail.
No doubt seeking to stay clear of producing a string of damaging publicity, Google claims that it took pains to mitigate biases in MUM — primarily by instruction the model on “high quality” information and getting humans evaluate MUM’s search outcomes. “We use [an] evaluation process to look for problems with bias in any set of applications that we launch,” Nayak stated. “When we launch things that are potentially risky, we go the extra mile to be extra cautious.”